The Ultimate Guide to Video Labeling Tools for Enhanced Data Annotation
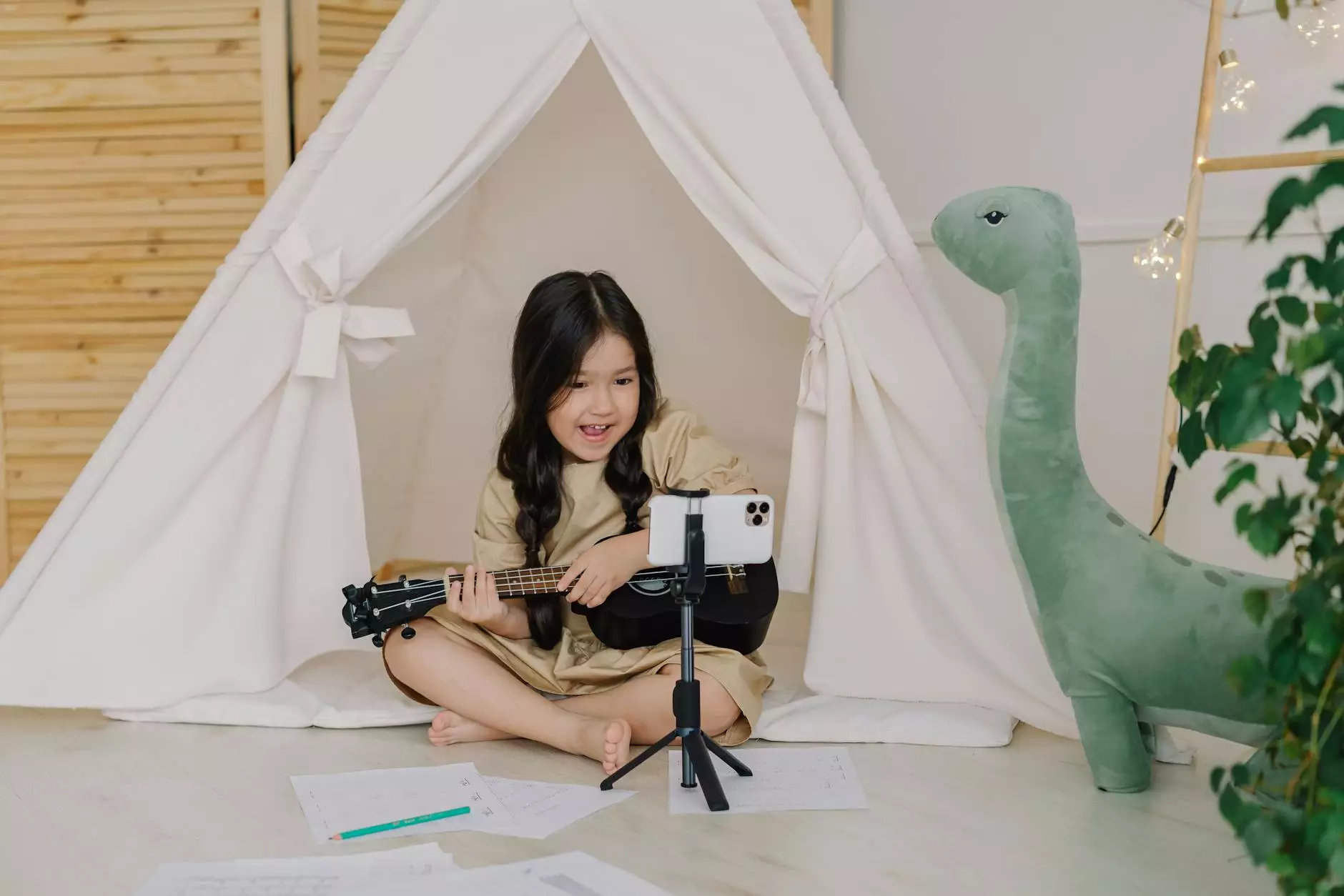
In today’s digital world, effective data management and annotation are imperative for businesses looking to leverage artificial intelligence (AI) and machine learning (ML). One significant area of focus is the creation and utilization of a video labeling tool to streamline data annotation processes. This article delves into the world of video labeling tools, their importance in data annotation, and how they can revolutionize your business operations.
What is a Video Labeling Tool?
A video labeling tool is software that enables users to annotate video data efficiently. This tool is essential in various fields including autonomous driving, healthcare, and security, where large amounts of video data need to be processed and labeled for AI training. The primary function of these tools is to allow users to categorize, tag, and organize segments of video content, making it easier for machine learning algorithms to learn from them.
Key Features of Video Labeling Tools
- Intuitive User Interface: A user-friendly interface that simplifies the annotation process for both experts and novices.
- Support for Multiple Video Formats: Capability to handle various video formats ensuring flexibility and usability.
- Collaborative Features: Enables multiple users to work on the same project simultaneously, enhancing productivity.
- Advanced Annotation Options: Supports various annotation types such as bounding boxes, polygons, and segmentation masks.
- Integration with Machine Learning Frameworks: Ability to export data in formats compatible with popular ML frameworks.
The Importance of Video Annotation in Data Annotation
Video annotation plays a critical role in modern data annotation, particularly as industries increasingly rely on video data for AI development. The following points illustrate the significance of video annotation:
Enhancing AI Training
Quality annotated video data is essential for training robust and accurate AI models. By providing clear labels and classifications, businesses ensure that their AI systems learn effectively from diverse scenarios presented in the video. This leads to improved decision-making capabilities and more reliable AI outputs.
Facilitating Better Predictive Analytics
With detailed video annotations, organizations can boost their analytics capabilities. Annotated video data enables deeper insights into behaviors, trends, and anomalies—key factors that drive predictive analytics and strategic planning.
Improving Operational Efficiency
Utilizing a video labeling tool can drastically improve operational efficiency. By automating parts of the annotation process and enabling team collaboration, businesses can speed up data preparation times and allocate resources more effectively.
How to Choose the Right Video Labeling Tool
Selecting a suitable video labeling tool can be a daunting task, given the variety of options available. Here are some factors that should influence your decision:
1. Ease of Use
The interface of the tool should be user-friendly, allowing for quick onboarding and minimal training requirements. An intuitive design ensures that users can focus on the task of annotation rather than struggling with the software.
2. Scalability
Your chosen tool should be scalable to accommodate growing data needs. Consider tools that can handle an increasing volume of videos without compromising performance.
3. Customization Options
Different projects have varying requirements, so look for tools that offer customization in terms of annotation types and workflow management.
4. Integration Capabilities
Ensure that the tool can integrate seamlessly with other software systems you are using. This will enhance compatibility and streamline workflow processes.
5. Price vs. Value
While cost is an important factor, evaluating the overall value the tool provides is imperative. Sometimes investing in a more expensive tool can yield better long-term benefits through faster processing and higher quality output.
Best Practices for Video Annotation
1. Define Clear Objectives
Before starting your video annotation project, it is crucial to define clear objectives. Knowing what you want to achieve with the annotated data will guide the annotation process.
2. Use Consistent Labeling Guidelines
To maintain uniformity and minimize errors, develop and follow standardized labeling guidelines. This ensures that all team members are on the same page and the data remains coherent.
3. Regular Quality Checks
Implement regular quality checks throughout the annotation process. Having quality assurance measures in place can help catch errors early and maintain high data quality.
4. Provide Adequate Training
Invest time in training your team on how to use the video labeling tool effectively. Knowledgeable users will produce better-quality annotations and work more efficiently.
5. Gather Feedback and Iterate
Encourage team members to provide feedback about the annotation process and the tool itself. Use this feedback to improve the workflow and the use of the tool.
Challenges in Video Annotation
While video annotation is a valuable process, it does come with its own set of challenges:
1. Resource Intensive
The video annotation process can be very labor-intensive, requiring significant human resources and time, especially for lengthy videos.
2. Subjectivity in Annotation
Different annotators may interpret labeling guidelines differently, leading to inconsistencies in the data that can affect machine learning outcomes.
3. Complexity of Videos
Videos containing multiple objects, actions, or scenes can be complex to annotate. This complexity may necessitate more advanced tools and skilled annotators.
Future Trends in Video Annotation Technology
The field of video annotation is constantly evolving. Here are some anticipated trends that may shape the future:
1. Increased Automation
As algorithms become more sophisticated, we can expect higher levels of automation in video labeling. Automated tools may handle routine tasks, thereby freeing up human annotators for more complex work.
2. Integration of AI for Enhanced Annotation
Future video labeling tools may use AI to assist annotators, suggesting labels or enabling semi-automated annotation processes to speed up data preparation times.
3. Real-Time Annotation Capabilities
The incorporation of real-time annotation features could have profound implications for fields like security, where immediate analysis and labeling of video feeds is critical.
Conclusion
In conclusion, a video labeling tool is an invaluable asset in the world of data annotation. Not only does it streamline the annotation process, but it also significantly enhances the ability of businesses to train their AI systems effectively. By understanding its importance, choosing the right tool, and following best practices, businesses can gain a competitive edge in their respective industries. As technology evolves, embracing these tools and adapting to new trends will be essential for thriving in the rapidly changing landscape of data-driven decision-making.
If you're ready to elevate your video annotation processes, consider exploring the offerings at keylabs.ai. Transform your data annotation efforts today!