Mastering Machine Learning Data Labeling for Business Success
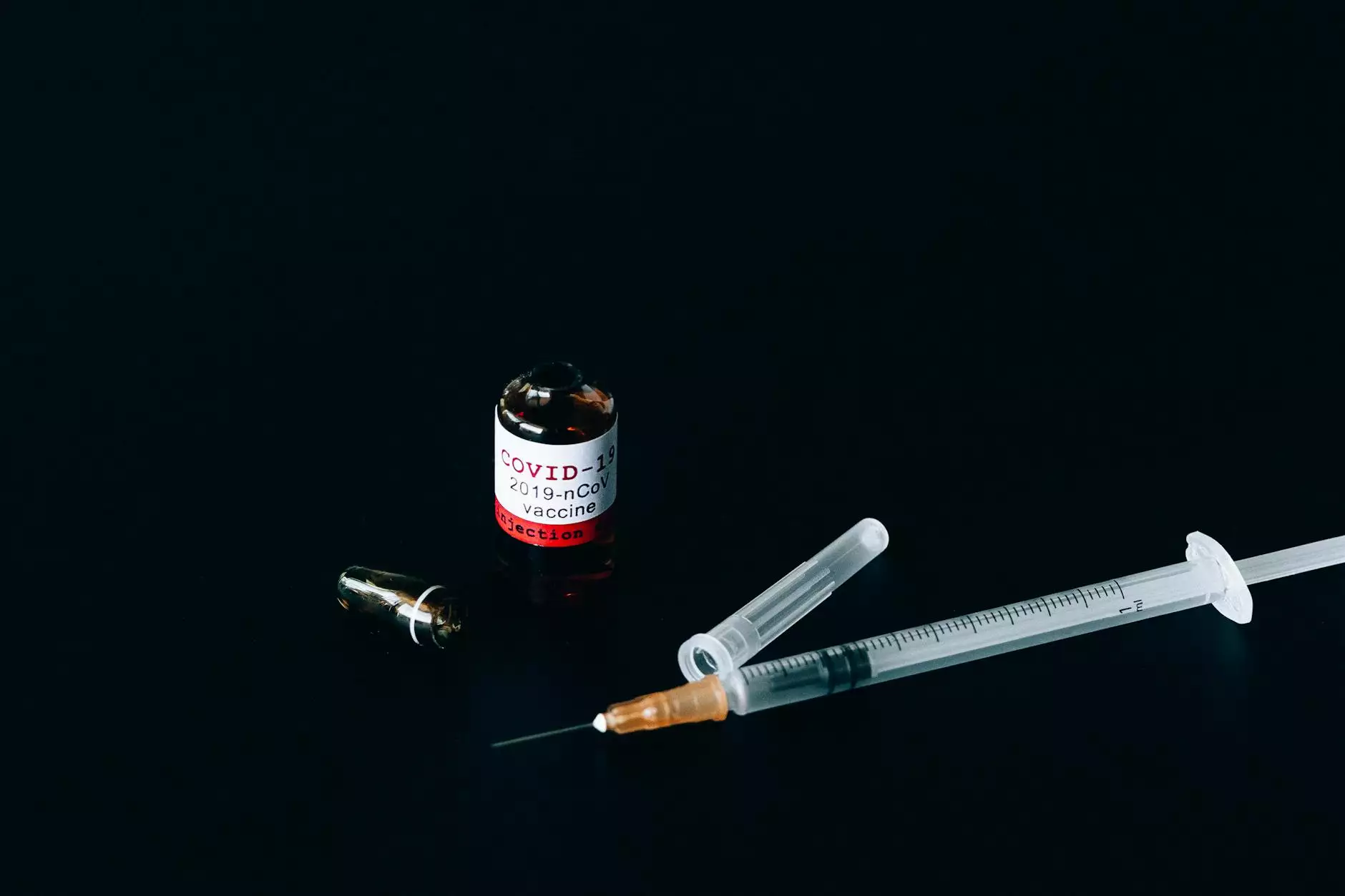
In today’s competitive landscape, businesses are increasingly turning to machine learning to enhance their operations, improve customer experience, and drive innovation. Central to the effectiveness of machine learning is a critical process known as data labeling. This article delves into the intricacies of machine learning data labeling, its significance in various industries, available tools and platforms, and its transformative impact on businesses.
What is Machine Learning Data Labeling?
Machine learning data labeling refers to the process of annotating or tagging data so that machine learning algorithms can learn from it effectively. The labeled data acts as a training set, enabling machines to recognize patterns, make predictions, and improve their accuracy over time.
Types of Data That Require Labeling
Almost any data can be labeled, but certain types are particularly significant for machine learning:
- Text Data: Sentiment analysis, chatbots, and document classification require labeled text data.
- Image Data: Applications such as facial recognition, object detection, and image segmentation rely on labeled images.
- Audio Data: Speech recognition systems and voice-assisted technologies need labeled audio samples.
- Video Data: Surveillance and event detection in video feeds rely on effective labeling for accurate real-time analysis.
The Importance of Accurate Data Labeling
Accurate machine learning data labeling is essential for several reasons:
- Improved Model Performance: High-quality labeled data leads directly to better-performing machine learning models, as the algorithms learn from accurate representations of the data.
- Efficiency in Training: Labeled datasets reduce the training time for algorithms, paving the way for faster implementation of AI solutions.
- Cost-Effectiveness: Reducing errors in prediction saves companies significant costs, both in resources and missed opportunities.
- Scalability: A well-labeled dataset scales better, as it can be reused for various applications without needing extensive rework.
Challenges in Machine Learning Data Labeling
While the advantages are compelling, businesses face numerous challenges when it comes to data labeling:
- Subjectivity: Different annotators may label the same data differently, leading to inconsistency and poor model performance.
- Volume of Data: In an era of big data, the sheer amount of data can overwhelm resources and make manual labeling impractical.
- Quality Assurance: Ensuring that labeled data meets a high-quality standard can be resource-intensive and difficult to manage.
Data Annotation Tools and Platforms
To effectively manage machine learning data labeling, businesses must leverage robust data annotation tools and platforms. Here are some notable options:
1. KeyLabs.ai
KeyLabs.ai provides an advanced data annotation platform that simplifies the labeling process, ensuring high-quality and efficient outcomes. Their user-friendly interface allows businesses to customize annotations based on their specific needs. KeyLabs.ai also offers tools for team collaboration, making it easier to maintain standards and consistency across projects.
2. Labelbox
Labelbox is another prominent data labeling platform that emphasizes collaboration and automation in the labeling process. With a focus on ease of use, it helps teams annotate data swiftly while maintaining quality through integrated review processes.
3. Supervisely
Supervisely caters to computer vision projects, offering tools for image and video annotation. Its comprehensive suite features functionalities like 3D point cloud annotation, which is ideal for industries like autonomous driving.
Implementing a Data Labeling Strategy
For businesses to reap the full benefits of machine learning data labeling, a strategic approach is crucial:
Step 1: Define Objectives
Start by clearly defining what the organization aims to achieve with machine learning. What problems will it solve? How will success be measured? Setting clear objectives will guide the entire data labeling process.
Step 2: Select the Right Tools
Choosing the appropriate data labeling tool is essential. Consider factors like ease of use, scalability, and the specific features that fit your project needs. Platforms like KeyLabs.ai are tailored for enterprise-level solutions.
Step 3: Develop Guidelines
Create a detailed guideline for annotators to ensure consistency across labeled data. This can include rules for classification, labeling nuances, and review procedures.
Step 4: Train the Annotators
It’s important to train your data annotators thoroughly. A well-trained team is likely to produce higher quality data, reducing the need for extensive revisions later. Consider simulated labeling exercises to hone their skills.
Step 5: Quality Control
Integrate a quality control process to review labeled data regularly. This can involve random sampling of labeled data, cross-checking by multiple annotators, or using automated integrity checks.
Step 6: Iterate and Improve
Data labeling is an iterative process. Continuously collect feedback and look for areas of improvement. As your machine learning models evolve, so too should your labeling strategies.
Real-World Applications of Machine Learning Data Labeling
The applications of machine learning data labeling are vast and varied across numerous industries:
1. Healthcare
In the healthcare sector, labeled data is critical for developing diagnostic tools and predictive analytics. For example, labeled imaging data can be used to train models that assist radiologists in identifying tumors.
2. Finance
Financial institutions utilize labeled data for fraud detection, risk assessment, and customer segmentation. By marking transaction data, they can build algorithms that distinguish between legitimate and fraudulent activities.
3. Retail
Retailers leverage labeled customer behavior data to enhance personalization strategies. By annotating data from customer interactions, they create targeted marketing campaigns that resonate with specific demographics.
4. Automotive
In the automotive industry, labeled data is indispensable for autonomous vehicles. Data from sensors and cameras is annotated to help self-driving systems understand their environment and make safe driving decisions.
The Future of Machine Learning Data Labeling
The future of machine learning data labeling appears promising, with advancements in technology continuing to evolve the landscape:
1. Automation
AI-driven annotation tools are beginning to automate parts of the labeling process. These tools can pre-label data, allowing human annotators to focus on refining and correcting labels.
2. Crowd Annotation
Crowdsourcing platforms are becoming increasingly popular for large-scale data labeling projects. They allow businesses to tap into a global workforce to annotate vast datasets quickly.
3. Improved Strategies
As machine learning algorithms advance, the strategies for data labeling will also improve, allowing for more adaptability and responsiveness to changing datasets and requirements.
Conclusion
Effective machine learning data labeling stands as a pillar of success for businesses aiming to leverage artificial intelligence. By understanding its significance, overcoming challenges with the right tools, and implementing strategic processes, organizations can unlock tremendous value from their data. As technology continues to evolve, staying abreast of new methodologies and tools will further enhance the capabilities of businesses in the era of big data and machine learning.
For more insights into data annotation tools and platforms, visit KeyLabs.ai.